Egencia Self-Service
Overview
Egencia, the B2B travel subsidiary of Expedia, helps 8 million global business travelers book and manage their trips all over the world. Competitors include Booking.com, Airbnb, Concur, and TripActions.
By executing a multi-pronged self-service strategy, our team saved the company $10M annually (7% of operating costs).
My role
Sr. Product Designer
The team
1 x Sr. Product Manager 1 x Researcher 1 x Data Analyst 6 x Engineers
Timing / Duration
2018 / 1 year (too slow!)
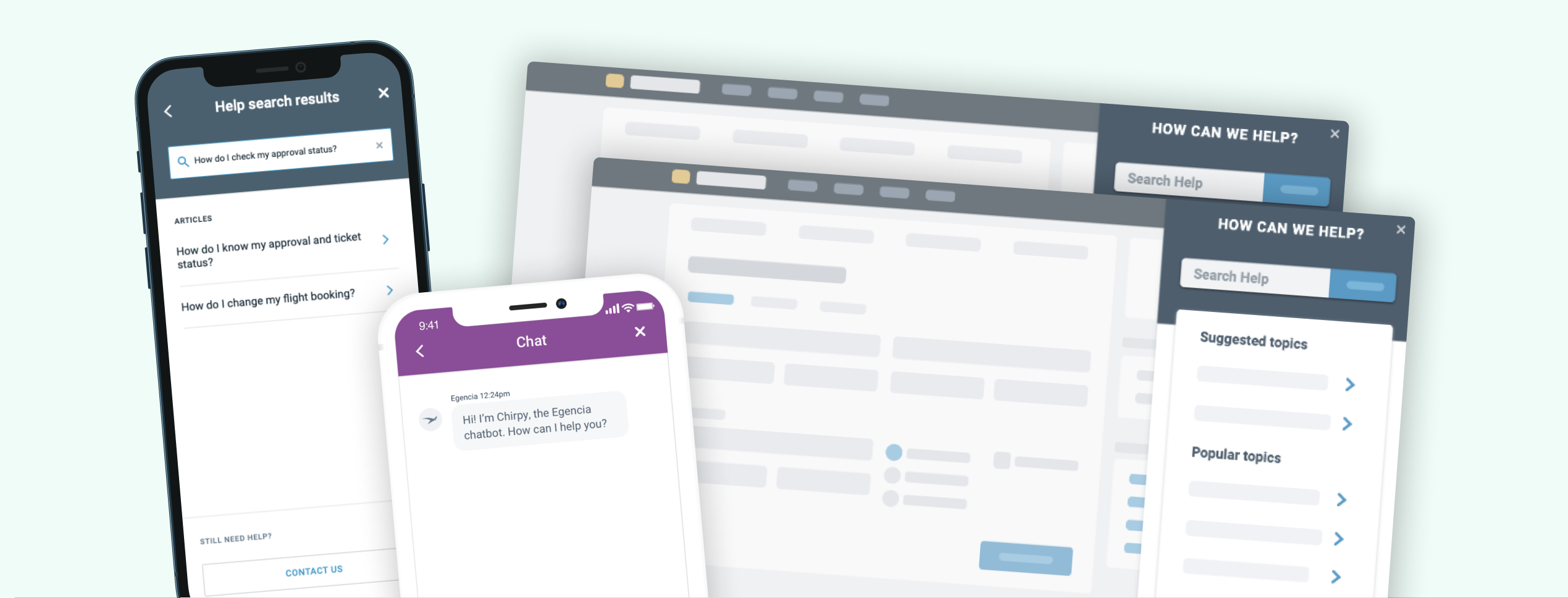
Background
In 2018, I was leading design on a cost-reduction team at Egencia. We testing across the product experience to educate, inform, and channel guide when a user needed help.
Our metrics ran in parallel to growth, which makes sense, because businesses increase the bottom line by 1) increasing revenue, or 2) decreasing expenses.
Executives challenged our team to reduce service calls, the highest operating expense in the company ($150M/year).

Nearly half 😱 of all travelers called Egencia at some point on their journey.
The Users
Personas were largely determined by job function and associated frequency of traveling. At a high level, these were categorized as the following:
- The Executive: 1-2 trips/month, booked by EA
- The Road Warrior: weekly to repeat locations
- The Annual Offsite Traveler: 1-3 trips/yr
- The Executive Assistant: booking for 1-3 execs
- The Bulk Arranger: booking company events (e.g. offsites)

The Strategy
To develop our strategy, we analyzed our goal through 4 lenses:
👥 What do our customers expect?
🏎️ What are our competitors doing?
🏗️ What are our team capabilities with current resourcing?
🌊 What does the company want/expect?
Objectives & Bets
We narrowed our efforts to three team objectives
- Educate Users
- Create an effortless journey
- Influence vertical product teams
We then prioritized six big bets that aligned to these objectives.
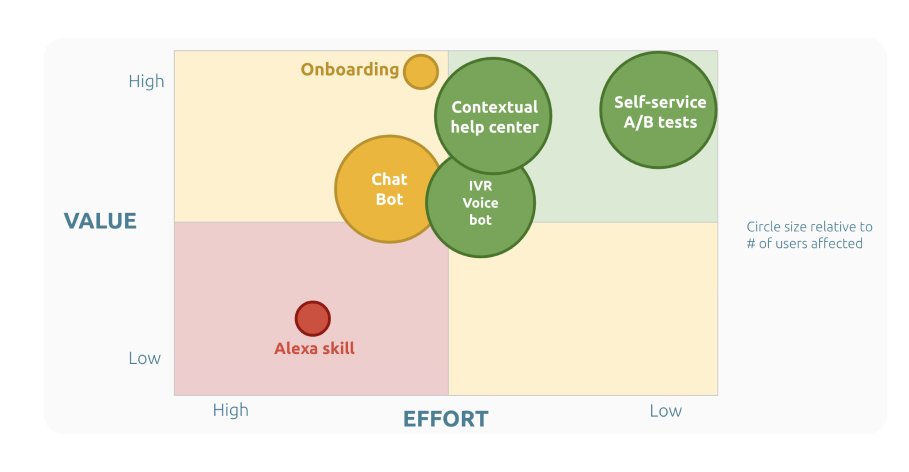
Note: For brevity, I'll focus this study on the contextual help center. View the full case study to go deep into the other big bets.
My Process
I audited products for inspiration, and tested competitor products.
It was a known issue that the help center was needing some product love. The content was relevant, having been refreshed by the marketing and customer support teams, yet the help center itself was merely a list of articles shown on a static page.
I wanted to redesign the help center to make the content more dynamic and timely, and I had a sense that making it contextual would be the ideal experience. Plus, it played well with the future idea of integration of a chatbot (see full case study).
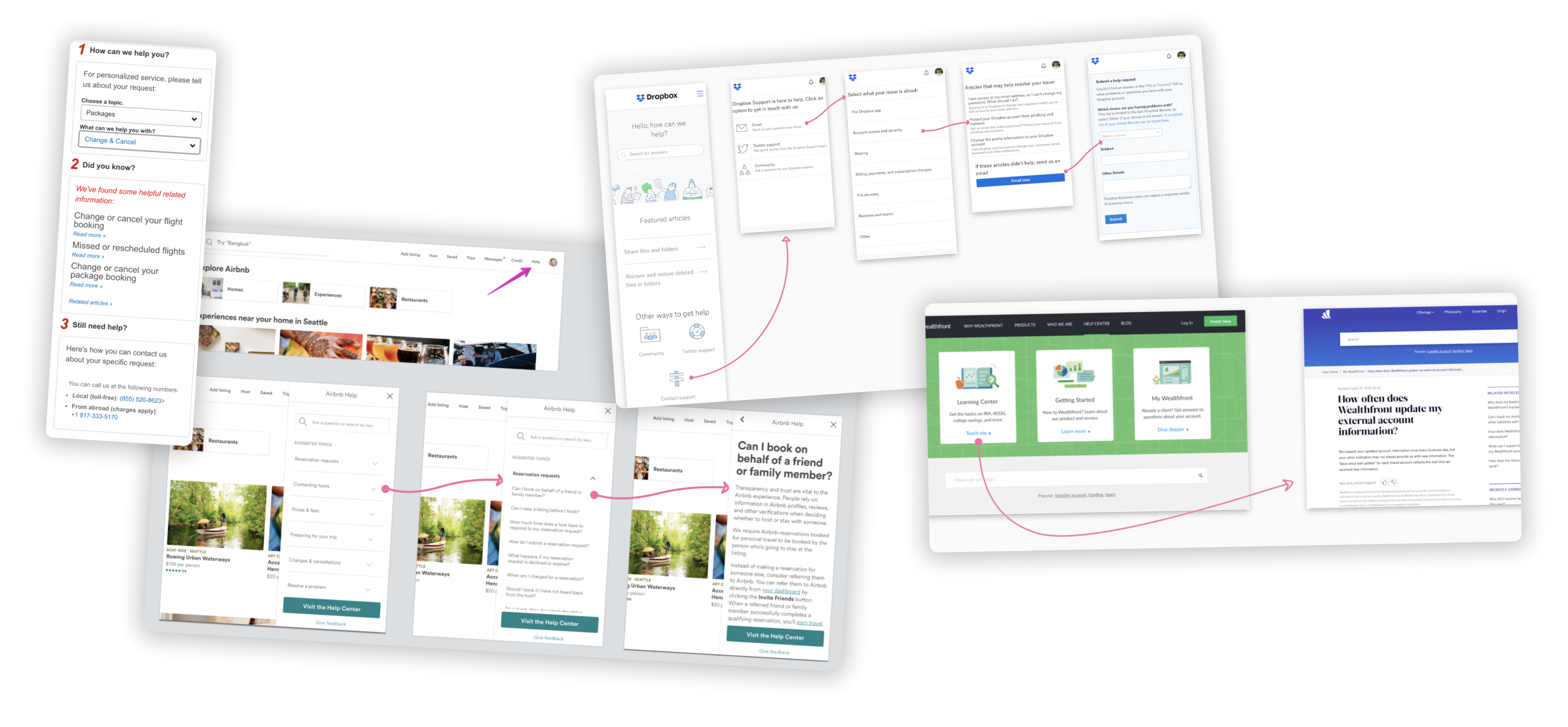
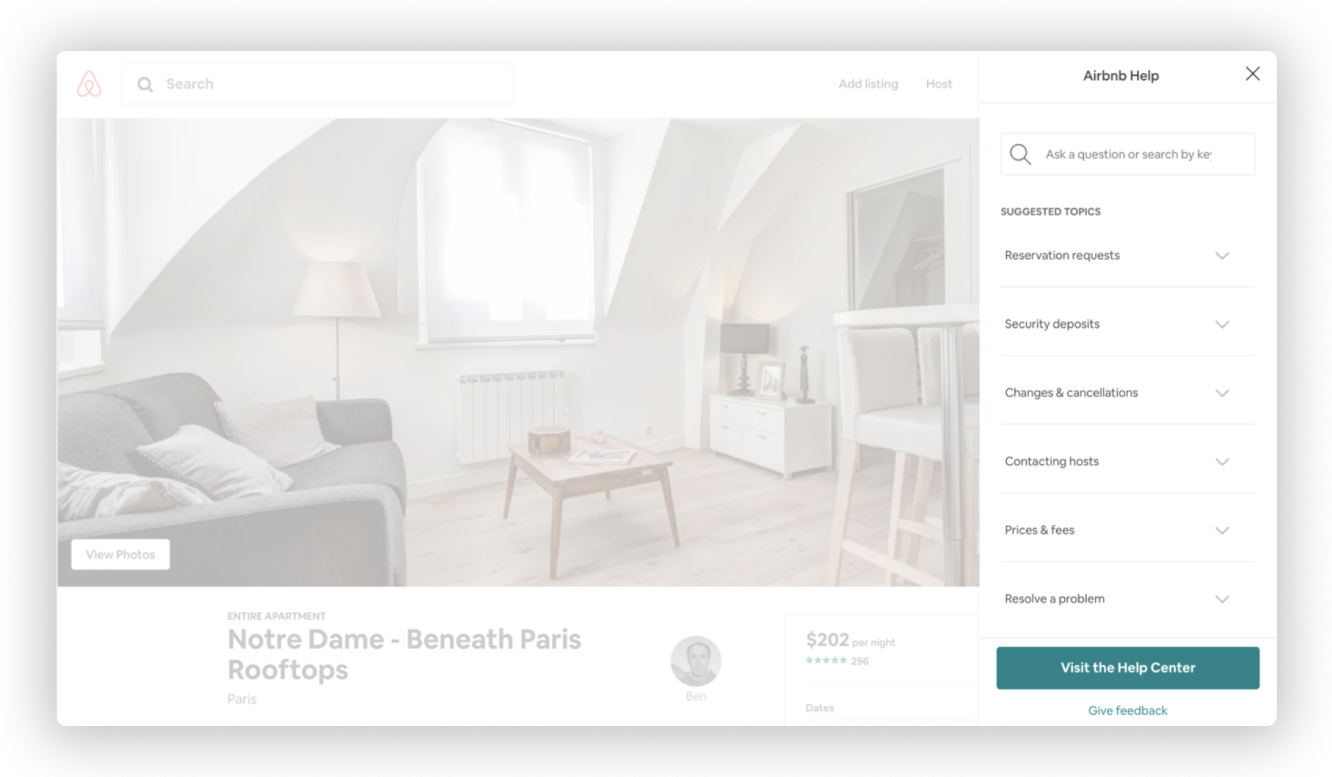
Airbnb's help center tested the best out of all the competitor tests I performed on usertesting.com.
Hypothesis
By creating a contextual help center that surfaces relevant help articles before showing contact options, users will self-serve without leaving their current flow, thereby decreasing call propensity and increase CSAT.
Solution-ing
To begin, I blockframed for quick feedback. I love this because it's quick, low-stakes, and fosters a collaborative atmosphere since everyone knows it's lower fidelity.
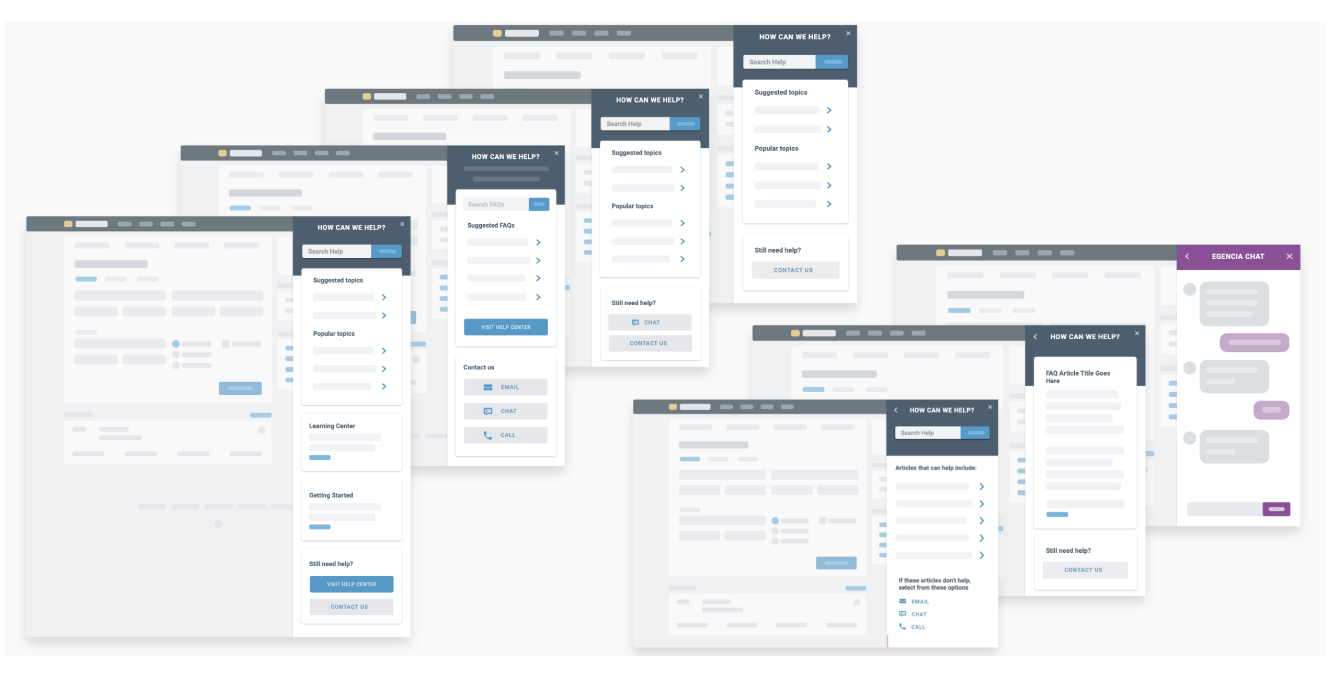
After a week of iteration filled with internal feedback from stakeholders and gathering constraints from engineers, I dove into higher fidelity options, testing three concepts via Usertesting.com.
Option 1: Channel Guidance
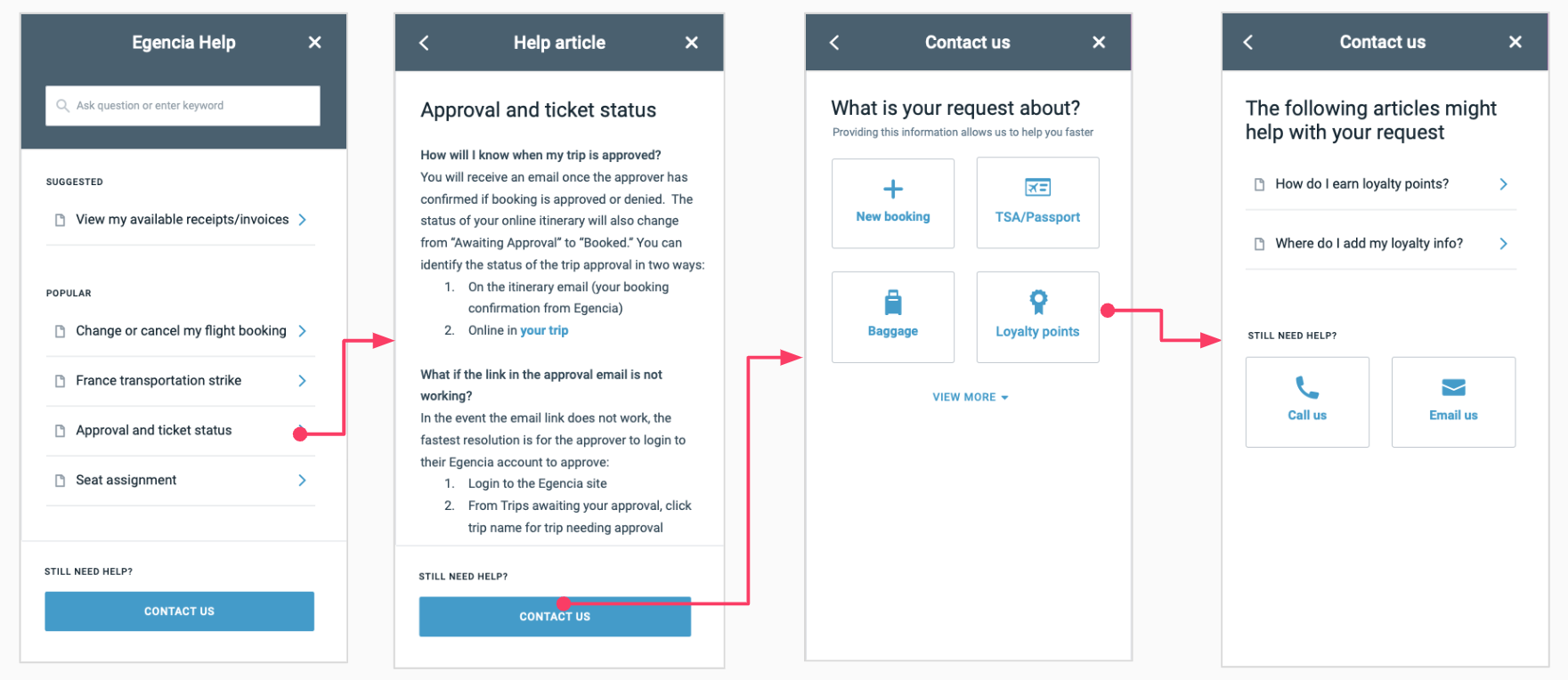
Option 2: Channel guidance + Trip
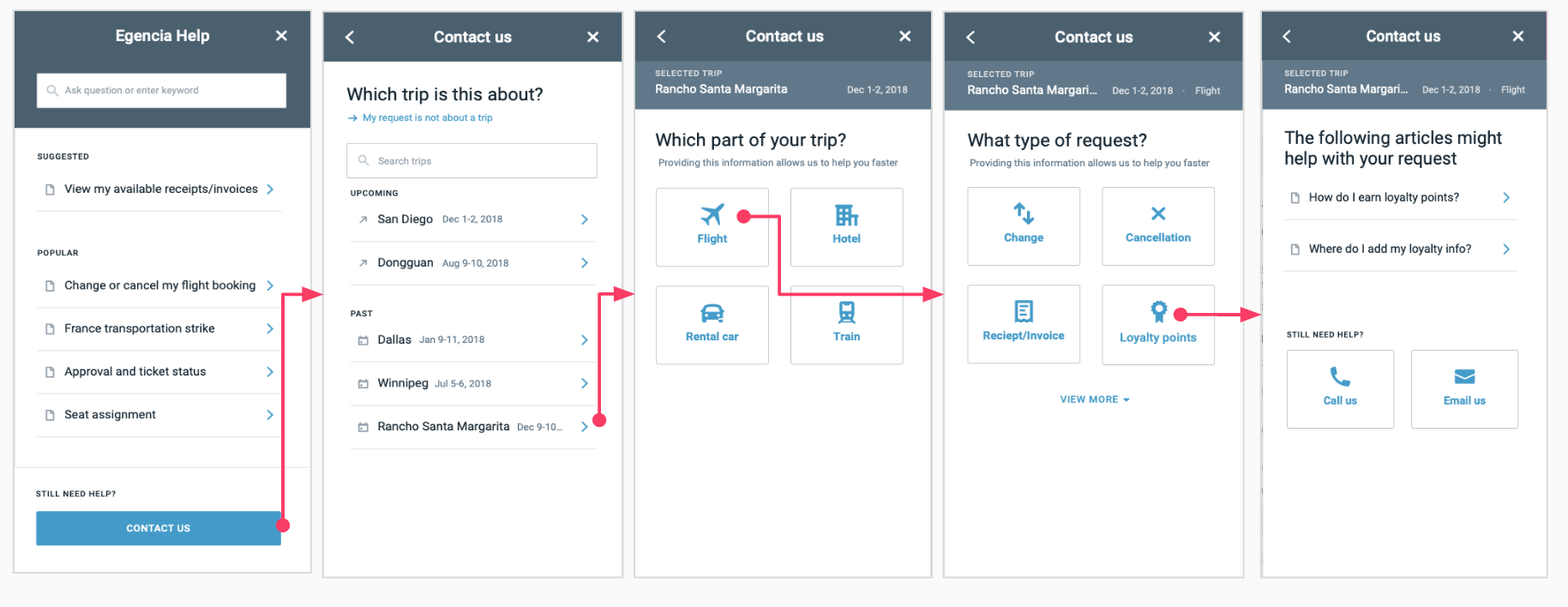
Option 3: Simple
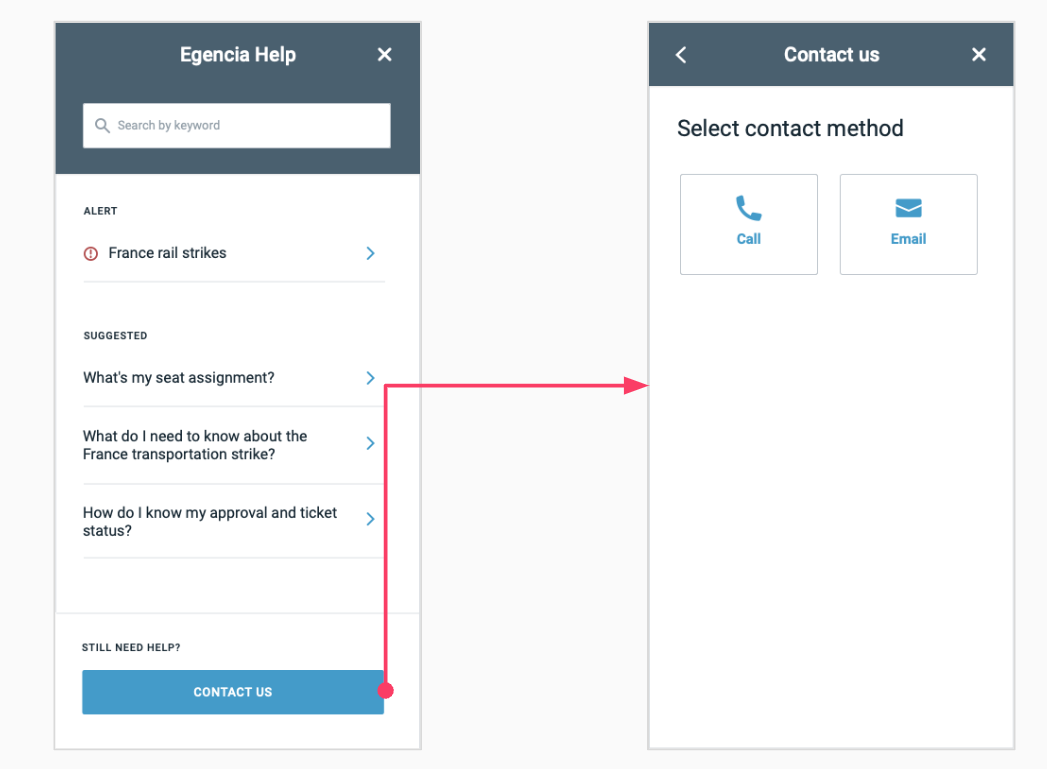
Unmoderated user testing results were positive for options 1 & 3.
Option 2 was too much friction for users. They expressed frustration since contact reasons didn't match their mental model. It have been the most beneficial option for the business because we could give them more targeted help articles with more input from users.
We didn't have the time to invest in more research, so we moved forward with option 3.
Option 3 Results
The test ran to statistical significance quickly. It had FAILED miserably. Calls went up by 5.9%. Hmmm 🧐
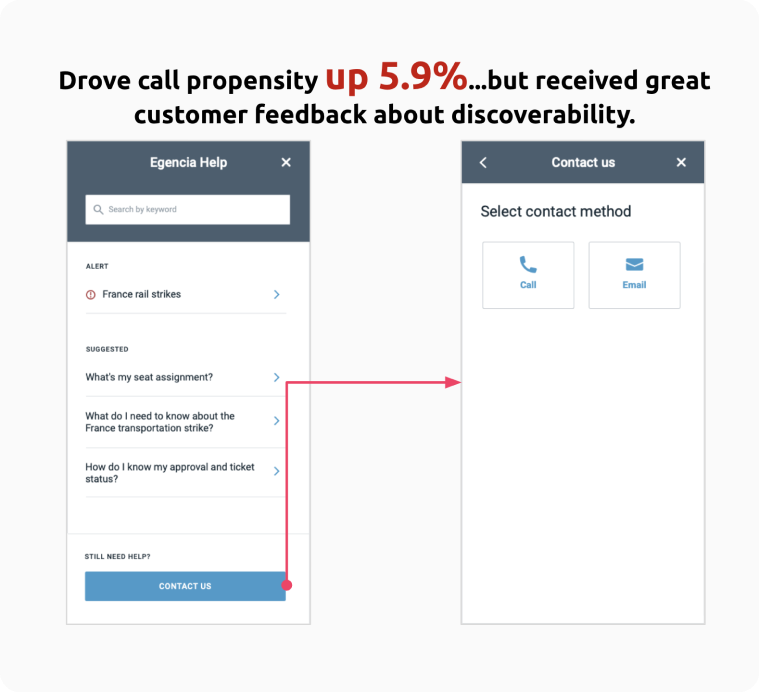
Final Test
I had suspected that Contact Us at the bottom of the page might be too discoverable. So, we decided to run a multivariant test:
- Variant A = Legacy contact flow
- Variant B = Reduced button prominence
- Variant C = Reduced button prominence + shown post search
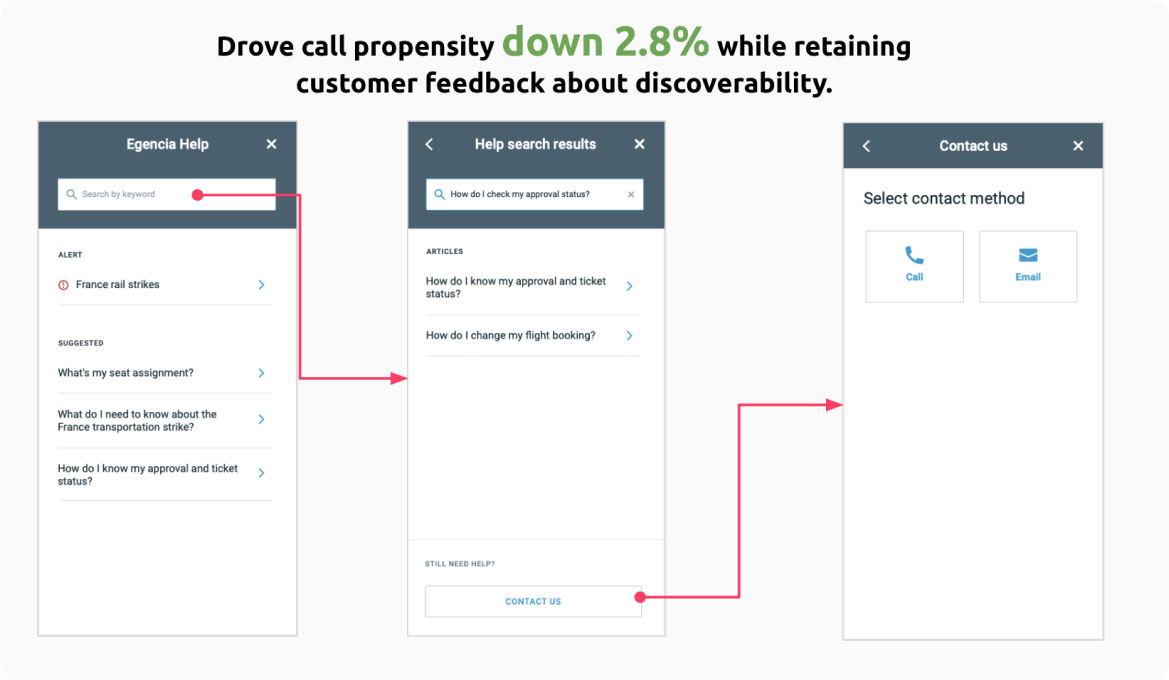
Final Results
Variant C lowered calls by 2.8% and did no harm to CSAT. It was a clear winner in this multivariant test. We rolled it out globally, saving $4.2M annually.
My Learnings
Aggressive testing = rapid learning
Executive leaders were conservative with our testing strategy. We were encouraged to test in smaller geographies with high CSAT, so as to not affect churn rates.
This reduced our bucket size of potential users, which led to long wait times to reach statistical significance. I grew tired of this cautious approach -- some of our tests ran for months!
My new motto is now: Ship frequently, measure often, learn quickly.
Keep it ethical AND practical
I had worried that Variant C was a borderline dark pattern, as it didn't feel ethical to bury Contact Us until after a search.
Variant C ended up doing no harm to customer sentiment, plus help article views went up. I was pleasantly surprised.
I learned to test patterns that may feel borderline dark...grey patterns? They might turn out to be just what the user AND business needs.